Quantitative Data Collection
Navanti has created representative samples for the quantitative surveys it conducts in fragile and conflict-affected areas since 2015. We employ our own trained field research teams in conjunction with local and third-party datasets and emergent geospatial technologies for subsequent data collection. Representative sampling is often a challenge due to missing or outdated data, population displacement, fluid conditions, and issues of access. While Navanti begins with census data or official population projections wherever possible, these are often insufficient, particularly in conflict zones and when sampling is needed at district or other local levels. Within northeastern Nigeria, Yemen, Tajikistan, Kyrgyzstan, southwestern Niger, and northern Burkina Faso, Navanti has employed field researchers to consult with local leaders and other key informants on the ground for current data on displacement, population distribution and access, and has used geofencing to map localized areas including formal and informal IDP settlements, informal neighborhood boundaries, and rural hamlets. This data is then triangulated with satellite imagery, gridded population density estimates, and displacement tracking datasets to create sample frames for multistage clustering using population proportional to size (PPS).
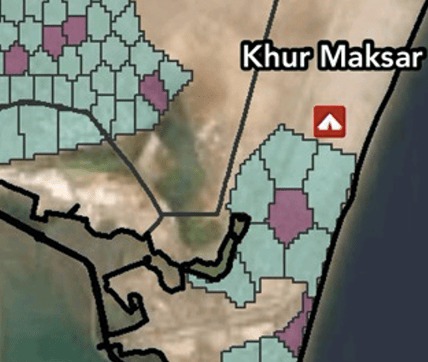
As with Navanti’s other approaches to field data collection, we adhere to best practices in ensuring that survey data collection:
- does no harm; upholds safety and comfort of participants
- enumerators, and others associated with fieldwork
- remains conflict- and gender-sensitive from design through execution
Survey instruments are regularly scoped and designed in collaboration with our clients and our field teams together, to:
- adapt needed data points and established scales to local contexts
- identify key vulnerable populations and set appropriate procedures for their inclusion
- introduce data collection to community stakeholders
- adapt results of pre-testing and piloting. Instruments are regularly translated and back-translated into relevant local languages, including discussion of sensitive and localized terms with field teams.
Remote and hybrid approaches to training, capacity building, management, and quality control are not new to Navanti. Since our founding, we have used emerging information technologies to prepare teams for the field and ensure data quality in numerous challenging environments, and quite often in locations with low or minimal digital connectivity. We have learned to adapt our training, field supervision, and quality control practices to whatever the conditions allow, from live video feed connecting stakeholders across the globe, to asynchronous learning and discussion with intermittent touchpoints. Above all, we work to develop the capacities of our colleagues in the field and develop reliable partnerships to adhere to the highest data collection standards.
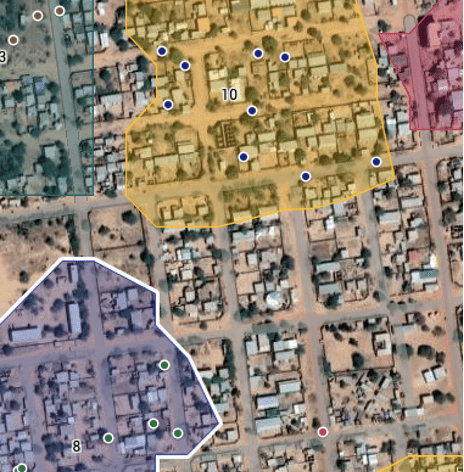
Understanding the need for trust and rapport between enumerators and participants in the brief interaction for data collection, Navanti has always sought to select enumerators from among members of communities chosen for data collection, so that they may be met by participating households and individuals as neighbors rather than outsiders. Just as definitions and meanings of community vary widely, enumerator selection applies considerations of gender, age, sexual orientation and gender identity and expression (SOGIE), ethnicity, and other markers of identity and belonging as appropriate to each study and its context. For survey data collection, gender is perhaps among the most important markers of identity, and Navanti understands that both gender-sensitivity and data quality requires same gender data collection (women interviewing women, and men interviewing men) in many cases depending on context and research topic. We approach every quantitative data collection effort as an opportunity to learn together with our colleagues in the field and improve our application of gender standards to the particular contexts of communities where we work – through our training, field supervision, quality assurance, and analysis.